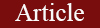
Machine Learning-Based Survival Prediction in Cancer Patients Treated with Nimotuzumab: A Real-World Data Mining Approach
Carmen Viada 1, Mayra Ramos-Suzarte 1, Lázara García 1, Aliuska Frias 1,
Yanela Santiestaban 1, Yuliannis Santiesteban 1, Mabel Alvarez 1, Marta Fors 2*,
Yanela Santiestaban 1, Yuliannis Santiesteban 1, Mabel Alvarez 1, Marta Fors 2*,
1 Center of Molecular Immunology/Havana/Cuba; carmen@cim.sld.cu; mayra@cim.sld.cu; lazarag@cim.sld.cu;aliuska@cim.sld.cu; yanela@cim.sld.cu; yula@cim.sld.cu;mabel@cim.sld.cu.
2 Universidad de Las Américas, Quito, Ecuador;
*Correspondecia: forsmarthamaria@gmail.com;
ABSTRACT
Nimotuzumab, a humanized monoclonal antibody targeting the epidermal growth factor receptor (EGFR), has demonstrated clinical benefit in various epithelial tumors. However, selecting the right patient populations remains a challenge, particularly when considering real-world treatment scenarios and diverse tumor types. This study aimed to identify clinical and demographic subgroups of patients with the highest survival benefit from Nimotuzumab, using decision tree models applied to integrated clinical trial data.
A total of 1,871 patients diagnosed with head and neck, brain, and esophageal cancers were included from 19 studies (phases I to IV and observational). Survival-related variables were analyzed using decision tree algorithms, a machine learning method suited for revealing complex, non-linear patterns among clinical features. The models stratified patients based on baseline characteristics and treatment outcomes.
The results revealed distinct predictive profiles for each cancer type. In head and neck cancer, survival was best predicted by race, disease status, use of radiotherapy, performance status, and toxic habits. In brain tumors, the most influential variables were age, performance status, and histological subtype. In esophageal cancer, survival was mainly determined by sex, histological diagnosis, and race. These variables consistently emerged as key decision nodes in the trained models.
The novelty of this study lies in its use of a machine learning approach across multiple tumor types treated with the same targeted therapy, Nimotuzumab. It is also among the first to apply such methodology to real-world data from low- and middle-income countries. By generating interpretable decision trees, the study offers a practical tool for identifying patient subgroups that are most likely to benefit from EGFR-targeted treatment, thereby supporting more efficient, personalized cancer care.
These findings reinforce the value of integrating data mining and artificial intelligence techniques into oncology to enhance treatment selection and improve patient outcomes.
Keywords: Nimotuzumab, EGFR-targeted therapy, Real-world data, Survival prediction, Machine learning in oncology, Decision tree analysis, Personalized cancer therapy, Head and neck squamous cell carcinoma, Glioma, Esophageal cancer, Biomarker-driven treatment, Clinical trial data mining, AI-based patient stratification, EGFR monoclonal antibody comparison, Clinical trial data integration
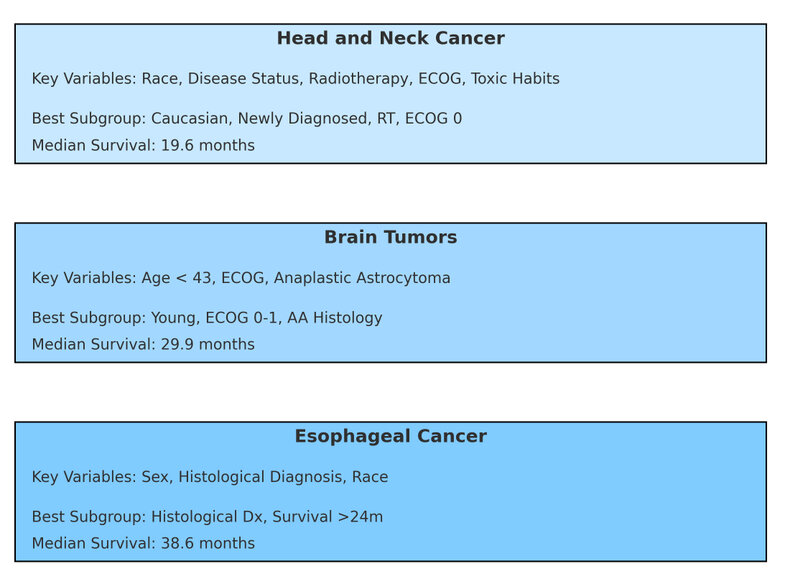
Figure Abstract. Simplified visualization of decision tree models applied to 1,871 patients across three tumor types: head and neck cancer, brain tumors, and esophageal cancer. Each panel shows the key predictive variables identified by the model, the subgroup with the highest median survival, and the corresponding survival outcome. These findings illustrate the value of AI-driven stratification to guide personalized treatment with Nimotuzumab in diverse clinical settings, especially in real-world and resource-constrained environments.
INTRODUCTION
Decision trees (DTs) are a supervised learning technique categorized as non-parametric and used for tasks like classification and regression. Their purpose is to build a model that predicts the value of a target variable by applying straightforward decision rules derived from the dataset's features. Essentially, a decision tree functions as a stepwise constant approximation. Structurally, it resembles a flowchart, where internal nodes correspond to attribute-based tests (e.g., whether a coin flip results in heads or tails), branches represent test outcomes, and leaf nodes indicate class labels or final decisions after evaluating all attributes. To better predict the cancer patients' survivability, only seven to ten features are identified from the available features as necessary for the analysis.
The epidermal growth factor receptor (EGFR) is a transmembrane protein that regulates cell proliferation and maturation, primarily in epithelial tissues such as the skin, intestinal mucosa, and liver. Two key ligands drive its activation: epidermal growth factor (EGF) and tumor growth factor alpha (TGF-α).1-2 These ligands trigger the EGF-EGFR signaling cascade by inducing receptor phosphorylation and dimerization. This system has been widely studied and is very attractive as a target for tumor therapy. It is associated with anarchic proliferation, cell immortalization, inhibition of apoptosis, neoangiogenesis, and metastasis: signs of poor prognosis that cause resistance to conventional oncological treatments, such as radiotherapy, chemotherapy, and hormonotherapy.3-4 Passive immunotherapy using monoclonal antibodies (MAbs) is among the most effective strategies targeting EGFR. There are currently several MAb against this receptor registered for the treatment of some epithelial solid tumors: Cetuximab® (chimeric MAb), Nimotuzumab (humanized MAb), and Panitumumab® (human MAb).5-6 Nimotuzumab is the international generic name for the drug, which is marketed under various brand names worldwide: Theraloc® in the European Union, TheraCIM® in Canada, Indonesia, and other Asian countries, CIMAher® in Cuba and Latin America, and BIOMAb-EGFR® in India.
The Molecular Immunology Center has been dedicated to the introduction of new products for the treatment of cancer. One of the products studied is Nimotuzumab in patients with glioma, head and neck, and esophageal cancer. When a product is registered in a new indication, knowing which patients benefit most is essential. Scientific problem: find subsets of patients treated with Nimotuzumab with the highest survival rate with this product.
This article aims to predict the best survival according to the patient's baseline characteristics and the use of Nimotuzumab.
MATERIAL AND METHODS
Decision tree learning, widely used in data mining7, aims to develop a model capable of predicting the value of a target variable based on multiple input variables. In this context, decision trees are seen as a blend of mathematical and computational methods that facilitate a dataset's description, classification, and generalization. The target variable, YYY, represents the dependent variable we seek to analyze, classify, or generalize, while a feature vector comprising x1, x2, x3, ..., xk, etc contains the input variables used for the task.
In data mining, decision trees are generally categorized into two types: classification trees, which predict the class (a discrete category) of the data, and regression trees, which indicate a continuous value (e.g., house prices or hospital stay durations). Additionally, some methods, known as ensemble techniques, create multiple decision trees. An example is boosted trees, which iteratively build an ensemble by focusing on training instances that were previously misclassified. A well-known example of this approach is AdaBoost.8 These can be used for both regression-type and classification-type problems. Another method is committees of decision trees (also called k-DT)9, an early approach to randomized decision tree algorithms that involved generating multiple trees from the training data and combining their outputs through majority voting. Bootstrap aggregation (or bagging), one of the first ensemble methods, creates numerous decision trees by repeatedly resampling the training data with replacement and using the aggregated votes for consensus predictions. The rotation forest method trains each decision tree by first performing principal component analysis (PCA) on a randomly selected subset of the input features.
Prominent decision tree algorithms include ID3 (Iterative Dichotomiser 3), its successor C4.5, and CART (Classification and Regression Tree). While regression and classification trees share similarities, they differ in aspects, such as the method for determining split points. OC1 (Oblique Classifier 1) was the first algorithm introducing multivariate splits at each node. Chi-square Automatic Interaction Detection (CHAID) performs multi-level splits for classification tasks. MARS extends decision trees to more effectively handle numerical data. Conditional Inference Trees employ a statistical approach, using non-parametric tests as splitting criteria with adjustments for multiple testing to minimize overfitting. This method ensures unbiased predictor selection and eliminates the need for pruning.
Before, the population was separated into two training samples (70%) and a validation sample (30%). After this, the classification tree was applied to both samples. Finally, the results of each subset were compared with those of the full population.
Head and neck cancer
For this study, 1110 patients with head and neck cancer were evaluated and included in ten clinical trials: three Phase I with 34 patients, two Phase II with 126 patients, one Phase III with 106 patients, two Phase IV with 632 patients, one observational study with 195 patients and one investigator-sponsored study with 17 patients (Table 1).
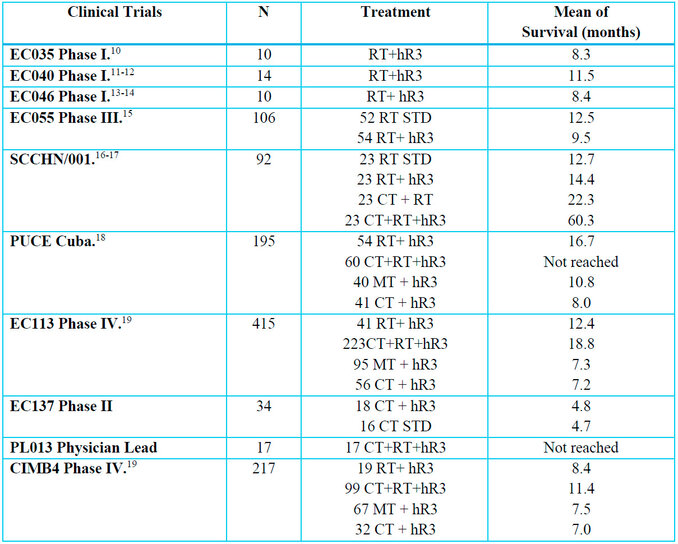
Table 1. Clinical trials in patients with head and neck cancer
Brain tumors
For this study, 405 patients with brain tumors were evaluated and included in five clinical trials: Phase II with 29 patients, Phase III with 70 patients, Phase IV with 220 patients, and one observational study with 86 patients (Table 2).
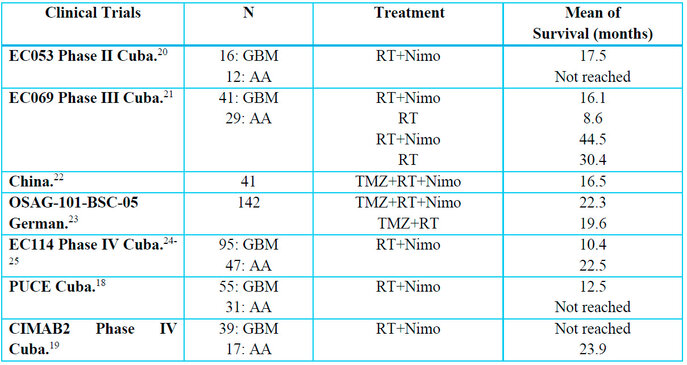
Table 2. Clinical trials in patients with brain tumors
Esophageal cancer
For this study, 356 patients with esophageal cancer were evaluated and included in four clinical trials: Phase II of 63 patients, three Phase III of 27, 107, and 160 patients, respectively (Table 3).
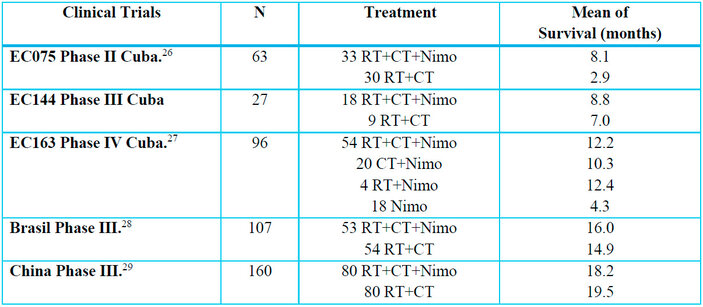
Table 3. Clinical trials in patients with esophageal cancer
RESULTS
Head and neck cancer
A decision tree was generated, taking as the dependent variable survival from inclusion and as independent variables the prognostic variables: Age, Sex, Race, Stage, Performance Status (WHO), Smoking, Alcohol, and Tumor Location. (Figure 1)
Figure 1. Decision tree for patients with head and neck cancer A) Training sample B) Validation sample C) Full sample. For a clearer view of Figure 1, it was accessed in an enlarged format on the website.
In the training sample, performance status was selected on the first level, race and radiotherapy were selected on the second level, and the toxic habit was selected on the third level (Figure 1 A). On the other hand, in the validation sample, radiotherapy was selected on the first level, and the toxic habit was chosen on the second level (Figure 1 B). However, in the entire population, race was selected on the first level, diseases were selected on the second level, and radiotherapy and performance status were selected on the third level (Figure 1 C). These are considered race, diseases, radiotherapy, performance status, and toxic habits are the primary outcomes for selecting the patients who benefit the most from head and neck cancer.
Brain tumors
A decision tree was constructed taking as the dependent variable, survival from inclusion, and as independent variables the prognostic variables: Age, Sex, Race, Karnosfky Incidence, Histological Type, Disease Diagnosis and Treatment Scheme. (Figure 2)
Figure 2. Decision tree for patients with brain tumors A) Training sample B) Validation sample C) Full sample. For a clearer view of Figure 2, it was accessed in an enlarged format on the website.
In the training sample, age was selected on the first level (Figure 2 A). On the other hand, in the validation sample, age was chosen on the first level, and the anatomopathological diagnosis was selected on the second level (Figure 2 B). However, in the full population, age was chosen on the first, performance status was selected on the second, and anatomopathological diagnosis was selected on the third (Figure 2 C). These are considered that age, performance status, and anatomopathological diagnosis are the main outcomes for selecting the patients who benefit the most in brain tumors.
Esophageal cancer
A decision tree was created, taking as the dependent variable survival from inclusion and as independent variables the prognostic variables: Age, Sex, Race, Stage, performance status, Histological Diagnosis, Toxic Habit, and Treatment Schedule. (Figure 3).
Figure 3. Decision tree for patients with esophageal cancer A) Training sample B) Validation sample C) Full sample. For a clearer view of Figure 3, it was accessed in an enlarged format on the website.
In the training sample, sex was selected on the first level, and histological diagnosis was chosen on the second level (Figure 3 A). On the other hand, in the validation sample, sex was selected on the first level (Figure 3 B). However, in the full population, sex was selected on the first level, histological diagnosis was chosen on the second level, and race was selected on the third level (Figure 3 C). These are considered that sex, histological diagnosis, and race are the primary outcomes for selecting the patients who benefit the most from esophageal cancer.
These decision trees allow us to predict the prognosis of survival for each cancer site evaluated according to the demographic and disease characteristics of each patient in each of the studies analyzed.
DISCUSSION
According to the authors, the past data mining trends used machine learning techniques and structured data stored in traditional databases. The current data mining trends use artificial intelligence and pattern reorganization techniques, as well as heterogeneous, semi-structured, and unstructured data. The future data mining trends used decision trees and neuronal networks and complex data, high-dimensional, temporal data, etc.30
The decision tree can handle both numerical and categorical data, but in implementation, we should try one hot encoding for categorical features before training or testing the model. Always keep in mind that the machine learning model understands nothing but numbers. Machine learning models use one hot encoding method to encode categorical variables as numerical values. It constructs new binary columns of every possible value from the original data.
Comparative Analysis: Nimotuzumab vs. Other EGFR-Targeted Therapies
EGFR-targeted therapies such as Cetuximab, Panitumumab, and Nimotuzumab have shown clinical benefits in various epithelial malignancies. However, important differences exist between them in molecular structure, efficacy profiles, safety, cost, and accessibility—particularly relevant factors in clinical decision-making and health policy.
Cetuximab is a chimeric monoclonal antibody (IgG1) approved for colorectal and head and neck cancers. It has demonstrated improvements in progression-free survival (PFS) and overall survival (OS) in combination with radiotherapy or chemotherapy 31. However, its use is often limited by high toxicity rates, particularly grade 3–4 skin rash, hypomagnesemia, and infusion-related reactions 32,33. Additionally, the high cost of Cetuximab makes it less accessible in low- and middle-income countries 34.
Panitumumab, a fully human IgG2 monoclonal antibody, offers reduced immunogenicity compared to Cetuximab and is approved for metastatic colorectal cancer with wild-type KRAS. Although it shares EGFR-blocking mechanisms, it has been associated with similar dermatologic toxicities and limited use outside of colorectal cancer 35. Panitumumab is also cost-prohibitive in many healthcare systems 36.
Nimotuzumab distinguishes itself by its favorable safety profile and lower incidence of severe adverse events, notably a significantly reduced rate of skin rash. This is attributed to its intermediate affinity for EGFR, allowing selective binding to overexpressed receptors on tumor cells while sparing normal tissues37, 38. Clinical studies have demonstrated meaningful survival benefits when combined with radiotherapy or chemoradiotherapy, particularly in head and neck squamous cell carcinoma and glioma 15,39. Moreover, Nimotuzumab has demonstrated economic advantages in cost-effectiveness and budget impact analyses conducted in Latin America 40,41. These findings suggest a higher accessibility potential in resource-limited settings.
From a regulatory perspective, Nimotuzumab has been approved in over 25 countries and marketed under various trade names (e.g., CIMAher®, TheraCIM®, BIOMAb-EGFR®), with particular penetration in low- and middle-income markets where alternatives like Cetuximab remain unaffordable or unavailable. Its integration into national treatment protocols in Cuba and India further reflects its strategic value in public health oncology programs42.
In summary, while all three anti-EGFR therapies target the same receptor, Nimotuzumab offers a unique balance between clinical efficacy, safety, and accessibility—making it a viable therapeutic alternative, particularly in settings where cost and toxicity are limiting factors.
Study benefits
The study provides specific information about which patients with head and neck, brain tumors, and esophageal cancer are most likely to benefit from Nimotuzumab. The results can help doctors make more informed and personalized patient treatment decisions. Including many patients in clinical trials of different phases increases the validity of the results. The use of decision trees facilitates the interpretation of results and their application in clinical practice. The fact that the study did not receive external funding increases its credibility, as there is no conflict of interest.
Two meta-analyses were conducted, one for Nimotuzumab in head and neck cancer patients and one for esophageal cancer patients, to evaluate the efficacy of Nimotuzumab in these locations.43-44 On the other hand, two studies were carried out, one on an economic evaluation and the other on the budgetary impact of Nimotuzumab on head and neck cancer.40-45
Limitations of the study
The study only considered a limited number of demographic and clinical variables that could be collected in most of the included studies. Other variables, such as the patient's overall health, could be included to provide more accurate results. The generated decision trees have a relatively low depth. More complex trees could be explored to identify additional patient subgroups that could benefit from Nimotuzumab. The study's results may be generalizable to patients from Cuba, China, Brazil, and Germany. The study results can only be generalized to cancer sites studied because each type of cancer has its specificities.
Additional data from the clinical trials included in the study and data from other sources could be analyzed to consider more variables and build more complex decision trees. Study results could be validated using data from different patient populations to assess their generalizability. This article proposes a working methodology to find patient niches that best benefit from nimotuzumab treatment in locations registered in Cuba and other countries, such as head and neck, brain tumors, and esophageal cancer.
CONCLUSIONS
This study demonstrates the value of using decision tree models to identify clinically relevant subgroups of cancer patients who derive the greatest survival benefit from treatment with Nimotuzumab. Based on real-world data from a large multicenter cohort of 1,871 patients with head and neck, brain, and esophageal cancers, we were able to define specific demographic and clinical profiles associated with better outcomes.
In head and neck cancer, the main predictive variables were race, disease status, radiotherapy use, performance status, and toxic habits. In brain tumors, the variables with the greatest discriminatory power were age, performance status, and histological diagnosis. For esophageal cancer, the most relevant predictors were sex, histological diagnosis, and race.
The novelty of this study lies in the use of a machine learning approach applied to integrated multicenter clinical trial data to build survival prediction models that can be translated into personalized therapeutic strategies. Unlike traditional survival analyses, the decision tree models used here are intuitive, easy to interpret, and provide concrete rules for stratifying patients based on real-world prognostic factors.
Another innovative aspect is the scope of the analysis: few studies have applied data mining methods across multiple tumor types for the same therapeutic agent, and even fewer in a low- and middle-income context where access to EGFR-targeted therapies is limited. This work therefore contributes to global oncology by offering a practical tool to optimize Nimotuzumab use where resources are constrained.
These results support the integration of machine learning techniques into oncological research and clinical decision-making, especially for patient selection in the use of targeted therapies like Nimotuzumab. Future studies with broader datasets and external validation are encouraged to refine and expand these models.
Author Contributions: Conceptualization, C.V. and M.F.; methodology, C.V., and M.F.; software, L.G., A.F. and M.A.; validation, C.V., Ya.S., and Yu.S.; formal analysis, C.V., and M.F.; investigation, C.V., and M.F.; resources, L.G., A.F. and M.A.; data curation, C.V., Ya.S. and Yu.S.; writing—original draft preparation, C.V.; writing—review and editing, M.F.; visualization, C.V.; supervision, M.F.; project administration, C.V.; funding acquisition, M.F. All authors have read and agreed to the published version of the manuscript.
Funding: This research received no external funding.
Institutional Review Board Statement: The study was conducted according to the guidelines of the Declaration of Helsinki and approved by the Institutional Review Board (or Ethics Committee) of the Cuban Regulatory Agency (CECMED) (protocol code and date of approval were provided in Materials and Methods).
Informed Consent Statement: Informed consent was obtained from all subjects involved in the study.
The patient(s) has obtained written informed consent to publish this paper.
Data Availability Statement: This section details where data supporting reported results can be found, including links to publicly archived datasets analyzed or generated during the study. Please refer to the suggested Data Availability Statements in the "Bionatura Research Data Policies" section at https://www.revistabionatura.com/policies.html. You might exclude this statement if the study did not report any data.
Acknowledgments: We can acknowledge any support not covered by the author's contribution or funding sections. This may include administrative and technical support or donations in kind (e.g., materials used for experiments).
Conflicts of Interest: The authors declare no conflict of interest. The funders had no role in the study's design; in the collection, analyses, or interpretation of data, the writing of the manuscript, or the decision to publish the results.
REFERENCES
1. Cohen, S. Isolation of a mouse submaxillary gland protein accelerating incisor eruption and eyelid opening in the new-born animal. J Biol Chem. 1962; 237:1555-62.
2. Cowley, G.P.; Smith, J.A.; Gusterson, B.A. Increased EGF receptors on human squamous carcinoma cell lines. Br J Cancer. 1986;53(2):223-9.
3. Ciardiello, F.; Tortora, G. Epidermal growth factor receptor (EGFR) as a target in cancer therapy: understanding the role of receptor expression and other molecular determinants that could influence the response to anti-EGFR drugs. Eur J Cancer. 2003;39(10):1348-54.
4. Mendelsohn, J.; Baselga, J. The EGF receptor family as targets for cancer therapy. Oncogene. 2000;19(56):6550-65.
5. Cohen, E.E. Role of epidermal growth factor receptor pathway-targeted therapy in patients with recurrent and/or metastatic squamous cell carcinoma of the head and neck. J Clin Oncol. 2006;24(17):2659-65.
6. Agulnik, M. New approaches to EGFR inhibition for locally advanced or metastatic squamous cell carcinoma of the head and neck (SCCHN). Med Oncol. 2012; 29(4):2481-91.
7. Rokach, L.; Maimon, O. (2014). Data mining with decision trees: theory and applications, 2nd Edition. World Scientific Pub Co Inc. doi:10.1142/9097. ISBN 978-9814590075. S2CID 44697571.
8. Hastie, T.; Tibshirani, R.; Friedman, J.H. (2001). The elements of statistical learning : Data mining, inference, and prediction. New York: Springer Verlag.
9. Heath, D.; Kasif, S.; Salzberg, S. (1993). k-DT: A multi-tree learning method. In Proceedings of the Second Intl. Workshop on Multistrategy Learning, pp. 138-149.
10. Rojo, F.; Gracias, E.; Villena, N.; Cruz, T.; Corominas, J.M.; Corradino, I.; et al. Pharmacodynamic trial of Nimotuzumab in unresectable squamous cell carcinoma of the head and neck: a SENDO Foundation study. Clin Cancer Res. 2010;16(8): 2474-82.
11. Crombet, T.; Rak, J.; Perez, R.; Viloria, A. Antiproliferative, antiangiogenic and proapoptotic activity of h-R3: A humanized anti-EGFR antibody. Int J Cancer. 2002;101(6):567-75.
12. Crombet, T.; Osorio, M.; Cruz, T.; Roca, C.; del Castillo, R.; Mon, R.; et al. Use of the humanized anti-epidermal growth factor receptor monoclonal antibody h-R3 in combination with radiotherapy in the treatment of locally advanced head and neck cancer patients. J Clin Oncol. 2004; 22(9):1646-54.
13. Crombet, T.; Pérez, R.; Lage, A.; Osorio, M.; Cruz, T. Anticuerpo monoclonal humanizado h-R3: un nuevo concepto terapéutico para el tratamiento del cáncer avanzado. Biotecnol Apl. 2003;20(1):33-51.
14. Crombet, T.; Torres, L.; Neninger, E.; Catala, M.; Solano, M.E.; Perera, A.; et al. Pharmacological evaluation of humanized anti-epidermal growth factor receptor, monoclonal antibody h-R3, in patients with advanced epithelial-derived cancer. J Immunother. 2003;26(2):139-48.
15. Rodriguez, M.O.; Rivero, T.C.; del Castillo, R.; Muchuli, C.R.; Bilbao, M.A.; Vinageras, E.N.; et al. Nimotuzumab plus radiotherapy for unresectable squamous-cell carcinoma of the head and neck. Cancer Biol Ther. 2010;9(5):343-9.
16. Ramakrishnan, M.S.; Eswaraiah, A.; Crombet, T.; Piedra, P.; Saurez, G.; Iyer, H.; et al. Nimotuzumab, a promising therapeutic monoclonal for treatment of tumors of epithelial origin. mAbs. 2009;1(1):41-8.
17. Babu, K.; Joseph, B.; Vidyasagar, M.S.; Bonanthaya, R.; Pasha, C.T.; Bapsy, P.P.; et al: An open-label, randomized, study of h-R3mAb (nimotuzumab) in patients with advanced (stage III or IVa) squamous cell carcinoma of head and neck (SCCHN): Four-year survival results from a phase IIb study [Abstract]. J Clin Oncol 2010;28(15 Suppl):428s.
18. Piedra, P.; Morejón, O. Report of the Fifth Nimotuzumab Global Meeting. Biotecnol Apl. 2010;27(1):56-61.
19. Piedra, P.; Saurez, G.; Barroso, M.; Ledón, N. Observational clinical study in patients with advanced stage epithelial tumors treated with Nimotuzumab [Abstract]. Can J Clin Pharmacol. 2010;17(1): e234.
20. Ramos, T.C.; Figueredo, J.; Catala, M.; Gonzalez, S.; Selva, J.C.; Cruz, T.M.; et al. Treatment of high-grade glioma patients with the humanized anti-epidermal growth factor receptor (EGFR) antibody h-R3: report from a phase I/II trial. Cancer Biol Ther. 2006;5(4):375-9.
21. Solomon, M.T.; Selva, J.C.; Figueredo, J.; Vaquer, J.; Toledo, C.; Quintanal, N.; et al. Radiotherapy plus the anti-EGFR mAb nimotuzumab or placebo for the treatment of high-grade glioma patients [Abstract]. J Clin Oncol. 2012;30(suppl):2515.
22. Hong, J.; Peng, Y.; Liao, Y.; Jiang, W.; Wei, R.; Huo, L.; et al. Nimotuzumab prolongs survival in patients with malignant gliomas: A phase I/II clinical study of concomitant radiochemotherapy with or without Nimotuzumab. Exp Ther Med. 2012;4(1):151-7.
23. Westphal, M.; Bach, F. Final results of a randomized phase III trial of Nimotuzumab for the treatment of newly diagnosed glioblastoma in addition to standard radiation and chemotherapy with temozolomide versus standard radiation and temoziolamide. J Clin Oncol. 2012;30(15 suppl 1):2033.
24. Saurez, G.; Cabanas, R.; Zaldivar, M.; Garnier, T.; Iglesias, B.; Piedra, P.; et al. Clinical experience with Nimotuzumab in cuban pediatric patients with brain tumors, 2005 to 2007. MEDICC Rev. 2009;11(3):27-33.
25. Saurez, G. Reporte del Séptimo encuentro científico internacional: Nimo–meeting 2012 en la sesión de tumores cerebrales. Rev Cubana Neurol Neurocir. 2012;2(2):171-6.
26. Ramos, M.; Luaces, P.; Lazo, N.G.; Perez, M.L.; Soriano, J.L.; Gonzalez, C.E.; et al. Treatment of malignant, non-resectable, epithelial origin esophageal tumours with the humanized anti-epidermal growth factor antibody nimotuzumab combined with radiation therapy and chemotherapy. Cancer Biol Ther. 2012;13(8): 600-5.
27. González, S.; Amador, Y.; Boris, L.G.; Mojena, L.; Soler, L.L.; Pish, G.; et al. Nimotuzumab in the treatment of inoperable esophagela tumors of epitelial origin. J. Oncol. 2022. Sep 1; 2022: 4128946. doi: 10.1155/20222/4128946. PMID: 36090901; PMCID: PMC 9458364.
28. Castro, G.; Segalla, J.; Stümoller, C.; Schmerling, R.; Andrade, C.J.; Pinczowski, H.; et al. Complete response (CR) to Nimotuzumab plus chemo-radiation in locally advanced esophageal cancer: Preliminary results of an ongoing randomized trial. Ann Oncol. 2010;21 Suppl. 8: viii260.
29. Feng, G.; Li, J.; Yu, N.; Zheng, Z.; Yang, X.; Deng, L.; et. al. Effectiveness and safety of combined Nimotuzumab and S-1 chemotherapy with concurrent radiotherapy for locally advanced esophageal cancer in mainourished and alderly patients: A prospective phase II study. Chin J Cancer Res. 2024 Jun 30; 36(3):270-281. doi: 10.21147/j.issn.1000-9604.2024.03.04. PIMD: 38988486; PMCID: PMC11230888.
30. Venkatadri, M.; Lokanatha, C.R. "A review on data mining from past to the future". International Journal of Computer Applications (0975-8887). Feb, 2011. 15(7): 19-22.
31. Bonner JA, et al. Radiotherapy plus Cetuximab for squamous-cell carcinoma of the head and neck. N Engl J Med. 2006;354(6):567–578.
32. Van Cutsem E, et al. Cetuximab and chemotherapy as initial treatment for metastatic colorectal cancer. N Engl J Med. 2009;360(14):1408–1417.
33. Cunningham D, et al. Cetuximab monotherapy and Cetuximab plus irinotecan in irinotecan-refractory metastatic colorectal cancer. N Engl J Med. 2004;351(4):337–345.
34. Johnson FM, et al. Cost effectiveness of Cetuximab for advanced HNSCC. Pharmacoeconomics. 2010;28(3):231–244.
35. Douillard JY, et al. Panitumumab-FOLFOX4 treatment and RAS mutations in colorectal cancer. N Engl J Med. 2013;369(11):1023–1034.
36. Geisler JP, et al. Economic comparison of Panitumumab and Cetuximab. Clin Ther. 2014;36(4):554–561.
37. Crombet T, et al. Use of the humanized anti-EGFR monoclonal antibody h-R3 in combination with radiotherapy. J Clin Oncol. 2004;22(9):1646–1654.
38. Ramos TC, et al. Treatment of high-grade glioma patients with h-R3: report from a phase I/II trial. Cancer Biol Ther. 2006;5(4):375–379.
39. Westphal M, et al. Nimotuzumab in glioblastoma: phase III results. J Clin Oncol. 2012;30(15 Suppl):2033.
40. Pérez, L.; Collazo, M.M.; Iznaga, N.; Viada, C.E. Evaluación económica del tratamiento con nimotuzumab para el cáncer de cabeza y cuello en Cuba. Vitae 24 (Supl. 1); 2017 S135
41. Pérez L, et al. Impacto presupuestal del nimotuzumab más quimiorradioterapia en pacientes con cáncer avanzado. Rev Cubana Oncol. 2021;19(2):e_136.
42. Ramakrishnan M, et al. Nimotuzumab: a promising therapeutic monoclonal for tumors of epithelial origin. mAbs. 2009;1(1):41–48.
43. Viada, C.; Vega, A.M.; Robaina, M. y col. Evaluación de Nimotuzumab para el tratamiento de cáncer de cabeza y cuello: Meta-análisis de ensayos controlados. Bionatura, Vol 5. No 1. 2020.
44. Viada, C.E.; Lima, M.; Soriano, J.L.; Fors, M.M.; y col. Meta-análisis de ensayos clínicos controlados de Nimotuzumab combinado con quimiorradioterapia en cáncer esofágico avanzado. Revista Cubana de Oncología. 2021 (Sep-Dic);19(3):e_148 ISSN 0864-0297
45. Pérez, L.; Soriano, J.L.; Rodríguez, M.M.; Soto, H.; Galán, Y.; Viada, C.E.; y col. Impacto presupuestal del Nimotuzumab más quimioradioterapia en pacientes con cáncer avanzado de cabeza y cuello. Revista Cubana de Oncología. 2021 (May-Ago);19(2):e_136 ISSN 0864-0297
Recibió: December,28 2024 / Aceptado : February, 25 2025 / Publicado: June 15, 2025
Citation: Viada, C.; Ramos-Suzarte M.; García, L.; Frias A; Santiesteban, Ya.; Santiesteban, Yu; Alvarez, M; Fors, M. Data mining in Nimotuzumab's database for different cancer. Bionatura journal. 2025;2 (2):7. doi: 10.70099/BJ/2025.02.02.7
Additional information Correspondence should be addressed: forsmarthamaria@gmail.com
Peer review information. Bionatura thanks anonymous reviewer(s) for their contribution to the peer review of this work using https://reviewerlocator.webofscience.com/
ISSN. 3020-7886
All articles published by Bionatura Journal are made freely and permanently accessible online immediately upon publication, without subscription charges or registration barriers.
Publisher's Note: Bionatura Journal stays neutral concerning jurisdictional claims in published maps and institutional affiliations.
Copyright: © 2025 by the authors. They were submitted for possible open-access publication under the terms and conditions of the Creative Commons Attribution (CC BY) license (https://creativecommons.org/licenses/by/4.0/).